The role of machine learning in wealth management
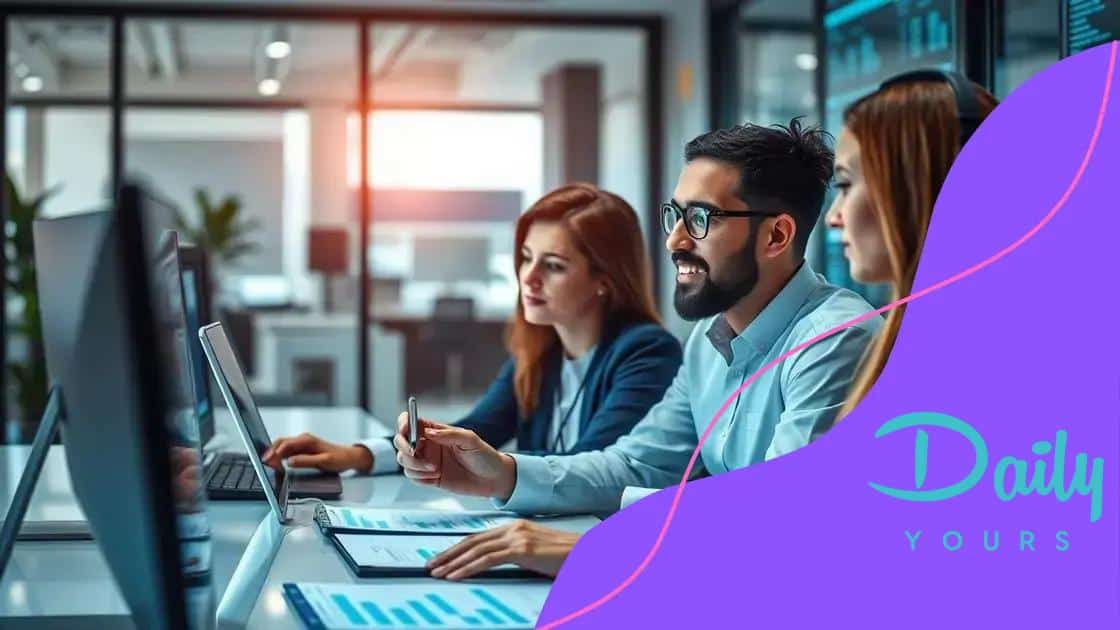
The role of machine learning in wealth management enhances decision-making, personalizes client experiences, automates processes, and addresses challenges related to data quality and system integration.
The role of machine learning in wealth management is changing the landscape of financial services. Have you noticed how technology is shaping investment decisions? Let’s dive into how this innovative approach enhances portfolio management.
Understanding machine learning in finance
Understanding machine learning in finance is crucial for grasping how modern technology shapes the industry. This technology helps financial professionals make better decisions and enhance their strategies.
What is Machine Learning?
Machine learning is the process where computers learn from data without being explicitly programmed. With this technology, systems can recognize patterns and make predictions based on input data.
Key Concepts in Machine Learning
Here are some fundamental concepts of machine learning that are important in finance:
- Algorithms: These are the mathematical models that help systems learn.
- Data Sets: Large volumes of historical data are used to train algorithms.
- Predictive Analysis: This technique forecasts future trends based on past data.
- Automation: Automating routine tasks saves time and reduces errors.
By understanding these concepts, financial professionals can leverage machine learning to optimize their wealth management processes.
Furthermore, the integration of machine learning in finance allows firms to analyze market trends quickly and efficiently. For example, algorithms can detect anomalies or risks in investment portfolios and alert managers in real-time. This quick response helps in making informed decisions that lead to better outcomes.
Moreover, machine learning enhances customer service through personalized financial products and advisory services. By analyzing a client’s financial history and preferences, technologies can suggest tailored solutions and strategies.
Key benefits for wealth management
Key benefits of machine learning for wealth management are transforming the way financial institutions operate. These advantages make it easier for firms to manage portfolios and meet client needs.
Improved Decision Making
One of the main benefits is enhanced decision-making. By analyzing large amounts of data, machine learning can identify trends and provide insights that humans might miss. This leads to more informed investment choices.
Increased Efficiency
Machine learning automates many routine tasks. This frees up time for financial advisors to focus on building client relationships and developing strategies. Automation reduces delays in processing transactions and improves overall service.
- Faster analysis: Data is processed quickly, allowing for real-time insights.
- Lower costs: Automation minimizes the costs related to manual tasks.
- Better client service: Advisors can dedicate more time to understanding clients’ needs.
- Enhanced reporting: More accurate reports are generated through automated systems.
Additionally, machine learning helps in identifying potential risks before they develop into bigger issues. Firms can use predictive analytics to foresee market fluctuations and adjust their strategies accordingly. By managing risks effectively, companies can protect their clients’ investments.
Personalization is another significant benefit. With the help of machine learning, wealth management can offer tailored financial products based on individual client profiles. This ensures that clients receive services that align with their unique financial goals and situations.
How firms are implementing ML solutions
Firms are increasingly adopting machine learning solutions to enhance their operational efficiency and client services. By integrating these technologies, they can stay competitive in a rapidly evolving financial landscape.
Integration into Existing Systems
Many organizations begin by integrating machine learning solutions into their existing systems. This allows them to leverage historical data, making the transition smoother. Existing databases can be used to train algorithms, which helps models become effective quickly.
Developing Custom Algorithms
Firms often develop custom algorithms tailored to their specific needs. By focusing on unique client requirements and market conditions, these algorithms can provide insights that generic solutions may miss. This customization makes the financial advice more relevant and timely.
- Data Collection: Gathering relevant data from multiple sources ensures algorithms have the necessary information.
- Testing and Validation: Firms rigorously test their algorithms to ensure accuracy and reliability before deployment.
- Continuous Improvement: Machine learning models are adjusted continuously based on new data and changing market dynamics.
- Training Staff: Proper training for employees is essential. Understanding how to use these tools helps maximize their benefits.
Moreover, organizations are investing in advanced analytics platforms to facilitate the implementation of machine learning. These platforms help in automating data processing tasks and generating actionable insights. As a result, firms can quickly respond to market changes and offer more accurate advice to their clients.
Additionally, collaboration with technology providers is common. By partnering with experts in machine learning, firms can access the latest innovations and enhance their capabilities, ensuring they remain at the forefront of the industry.
Challenges in adopting machine learning
Challenges in adopting machine learning in wealth management are significant but can be addressed with the right strategies. Understanding these hurdles is essential for firms looking to implement this technology successfully.
Data Quality Issues
One major challenge is the quality of data. Machine learning algorithms rely on large sets of accurate data. If the data is incomplete, outdated, or biased, it can lead to poor model performance. Firms must prioritize cleaning and organizing their data before using it.
Integration with Existing Systems
Integrating machine learning solutions with existing systems can also pose hurdles. Legacy systems may not be compatible with modern technologies. This can result in high costs and long implementation timelines.
- System Compatibility: Ensuring all systems work together smoothly.
- Scalability: Adapting solutions as data and business needs grow.
- Resource Allocation: Investing time and money into updating infrastructure.
- Staff Training: Upskilling employees to handle new technologies.
Moreover, there is often a lack of understanding about how machine learning works. Employees and management may have concerns about reliability and the unknowns associated with AI. This can hinder acceptance and slow down implementation processes.
Another challenge is regulatory compliance. The financial industry is highly regulated, and adopting machine learning must align with existing laws. Firms need to ensure their algorithms respect privacy and fairness standards to avoid legal issues.
Future trends in wealth management using ML
Future trends in wealth management using machine learning promise exciting developments that can improve client experiences. As technology evolves, we can expect several key changes in how financial services operate.
Enhanced Personalization
One major trend is enhanced personalization. With machine learning, firms can analyze vast amounts of data to understand client preferences better. This allows for tailored recommendations that align with individual financial goals.
Predictive Analytics
Another trend is the rise of predictive analytics. By predicting market trends, firms can provide proactive advice to clients. This means that investors can make better decisions based on anticipated market movements.
- Real-Time Insights: Clients will receive actionable insights that adjust with market changes.
- Risk Management: Predictive models will allow better risk assessment and management strategies.
- Customized Financial Products: Financial products will be designed specifically for each client’s needs.
- Automated Rebalancing: Portfolios will automatically adjust based on changing market conditions.
Moreover, machine learning will play a crucial role in improving operational efficiency. Automation of routine tasks will free up financial advisors to focus on building relationships with clients. As a result, advisory services will become more meaningful and effective.
Additionally, the integration of advanced technologies like artificial intelligence and big data will reshape how wealth management firms operate. These technologies will allow for more accurate forecasting and smarter investment strategies. As firms adopt these methods, they will enhance their competitive edge in the market.
In conclusion, the integration of machine learning in wealth management is reshaping the industry. By enhancing personalization, improving decision-making, and automating processes, financial firms can offer better client services. As challenges are addressed and technology evolves, the future of wealth management looks promising. Embracing these innovations will not only streamline operations but also strengthen client relationships, leading to greater success in the financial landscape.
FAQ – Frequently Asked Questions about Machine Learning in Wealth Management
What are the main benefits of using machine learning in wealth management?
The main benefits include enhanced personalization, improved decision-making, and greater efficiency through automation of processes.
What challenges do firms face when adopting machine learning solutions?
Firms face challenges such as data quality issues, integration with existing systems, and the need for staff training.
How does machine learning improve decision-making in finance?
Machine learning analyzes large datasets to identify trends and provide insights, facilitating better, data-driven investment decisions.
What future trends can we expect in wealth management using machine learning?
Future trends include enhanced personalization, predictive analytics for proactive advice, and improved operational efficiency through automation.